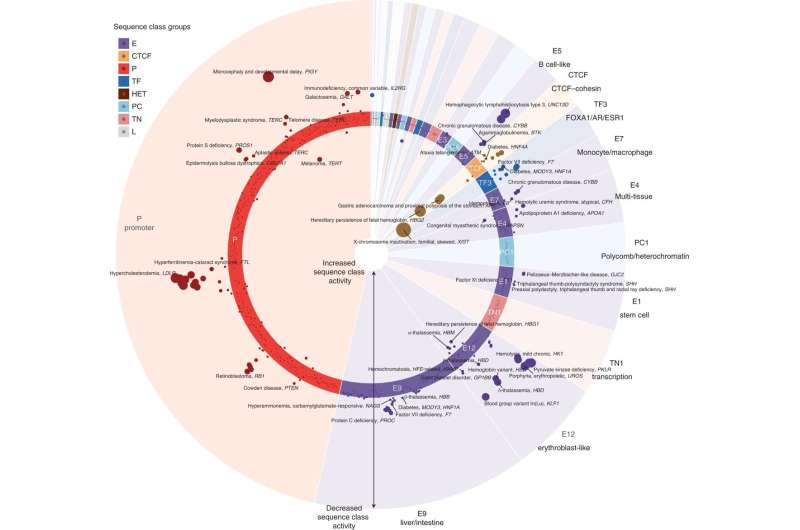
Disease regulatory mutations are predicted to disrupt promoter, CTCF, and tissue-specific enhancer sequence classes. Mutation effects at the sequence class level of pathogenic non-coding HGMD mutations were plotted. A polar coordinate system was used, with the radial coordinate indicating the class-level effects of the sequence. Each dot represents a mutation, and mutations within the circle are expected to have beneficial effects (increased sequence class activity); Mutations outside the circle are expected to have adverse effects (decreased sequence class activity). The point size indicates the absolute value of the effect. Mutations have been assigned to sequence classes based on their sequences and predicted effects. Within each sequence class, mutations were ordered according to chromosomal coordinates. The assigned disease and gene name were annotated for each mutation, and only the strongest mutation was annotated when multiple mutations were associated with the same disease, gene, and sequence class. Recognition: natural genetics (2022). DOI: 10.1038/s41588-022-01102-2
Newly developed artificial intelligence (AI) programs have accurately predicted the role of the regulatory elements and three-dimensional (3D) structure of DNA based solely on its raw sequence, according to two recent studies in natural genetics. These tools could eventually shed new light on how genetic mutations lead to disease and lead to a new understanding of how genetic sequence affects the spatial organization and function of chromosomal DNA in the cell nucleus, said study author Jian Zhou, Ph.D. , Assistant Professor in the Lyda Hill Department of Bioinformatics at UTSW.
“Taken together, these two programs provide a more complete picture of how changes in DNA sequence, even in non-coding regions, can have dramatic effects on its spatial organization and function,” said Dr. Zhou, a member of the Harold C. Simmons Comprehensive Cancer Center, a Lupe Murchison Foundation grantee in medical research, and a grantee from the Cancer Prevention and Research Institute of Texas (CPRIT).
Only about 1% of human DNA encodes instructions for making proteins. Research over the last few decades has shown that much of the remaining non-coding genetic material contains regulatory elements—such as promoters, enhancers, silencers, and insulators—that control how the coding DNA is expressed. How the order controls the functions of most of these regulatory elements is not well understood, explained Dr. zhou
To better understand these regulatory components, he and colleagues at Princeton University and the Flatiron Institute developed a deep learning model called Sei, which neatly sorts these snippets of non-coding DNA into 40 “sequence classes” or jobs—for example, as enhancers for gene activity of stem cells or brain cells. These 40 sequence classes, developed from nearly 22,000 data sets from previous studies studying genome regulation, cover more than 97% of the human genome. In addition, Sei can score each sequence based on its predicted activity in each of the 40 sequence classes and predict how mutations affect such activities.
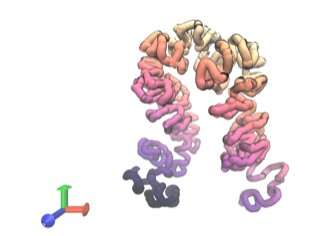
Predicted 3D structure for a segment of human genomic DNA. Photo credit: UT Southwestern Medical Center
By applying Sei to human genetic data, researchers were able to characterize the regulatory architecture of 47 traits and diseases recorded in the UK Biobank database and explain how mutations in regulatory elements cause specific pathologies. Such skills can help gain a more systematic understanding of how changes in genome sequence are related to diseases and other traits. The results were released this month.
In May, Dr. Zhou on developing another tool called Orca that predicts the 3D architecture of DNA in chromosomes based on their sequence. Using existing datasets of DNA sequences and structural data from previous studies that revealed the molecule’s folds, twists and turns, Dr. Zhou used the model to make connections and evaluated the model’s ability to predict structures at different length scales.
The results showed that Orca predicted both small and large DNA structures based on their sequences with high accuracy, including for sequences carrying mutations linked to various health conditions, including a form of leukemia and limb abnormalities. Orca also allowed researchers to develop new hypotheses about how DNA sequence controls its local and large-scale 3D structure.
dr Zhou said he and his colleagues plan to use Sei and Orca, both of which are publicly available on web servers and as open-source code, to further explore the role of genetic mutations in causing the molecular and physical manifestations of disease – Research This could eventually lead to new ways to treat these conditions.
A study reveals the “grammar” behind human gene regulation
Kathleen M. Chen et al, A sequence-based global map of regulatory activity to decipher human genetics, natural genetics (2022). DOI: 10.1038/s41588-022-01102-2
Jian Zhou, Sequence-based modeling of the three-dimensional genome architecture from the kilobase to the chromosome scale, natural genetics (2022). DOI: 10.1038/s41588-022-01065-4
Provided by UT Southwestern Medical Center
Citation: Artificial intelligence tools predict the regulatory role and 3D structure of DNA (2022 August 8) retrieved August 9, 2022 from https://phys.org/news/2022-08-artificial-intelligence-tools- dna-regulatory.html
This document is protected by copyright. Except for fair trade for the purpose of private study or research, no part may be reproduced without written permission. The content is for informational purposes only.
#Artificial #intelligence #tools #predict #regulatory #role #structure #DNA
Leave a Comment