The final product of the machine learning algorithm: metastable phase diagrams for carbon. The colored areas indicate conditions where carbon is in certain metastable states that can yield useful material properties. Photo credit: Argonne National Laboratory
A new computational approach will improve understanding of different carbon states and guide the search for yet-to-be-discovered materials.
Materials – we use them, wear them, eat them and create them. Sometimes we invent them by accident, like Silly Putty. But much more often, creating useful materials is a laborious and expensive process of trial and error.
Scientists at the US Department of Energy’s (DOE) Argonne National Laboratory recently demonstrated an automated process for identifying and researching promising new materials by combining machine learning (ML) – a type of artificial intelligence – and high-performance computing. The new approach could help accelerate the discovery and design of useful materials.
Using the single element carbon as a prototype, the algorithm predicted how atoms arrange themselves under a wide range of temperatures and pressures to form different substances. From there, it constructed a series of so-called phase diagrams — a kind of map that helps them find new and useful states of matter.
“We trained a computer to examine, question and learn how carbon atoms might be organized to create phases that we may not find on Earth or that we do not fully understand, and so a whole step in the materials evolution process automated,” said Pierre Darancet, an Argonne scientist and author of the study. “The more of this process a computer can do on its own, the more materials science we can do.”
balance and beyond
When the atomic structure of a material changes, its electronic, thermal, and mechanical properties also change. Scientists want to find new ways to arrange atoms to create useful materials. A fundamental way to change the atomic structure of a material is to vary the ambient pressure and temperature.
We often see this type of structural change in water. At room temperature and normal atmospheric pressure, water is the most stable liquid. If you lower the temperature far enough, the same water molecules arrange themselves into solid ice.
Similarly, diamond and graphite are entirely different materials, but both are made entirely of carbon atoms—just arranged in different ways. Under normal conditions, graphite is a much more stable form of carbon than diamond. However, under extreme conditions of pressure and heat, graphite slowly crystallizes into diamond. When removed from these extreme conditions, the diamond persists and dwells in what scientists call a metastable state.
The ML algorithm produced phase diagrams depicting hundreds of these metastable carbon states, some known and some new.
“It is experimentally difficult to predict and generate states of matter that are not near equilibrium conditions,” said Jianguo Wen, an Argonne experimenter on the study. “This new computational approach allows us to explore those little-known regions on the maps that are otherwise inaccessible or that we don’t even know exist.”
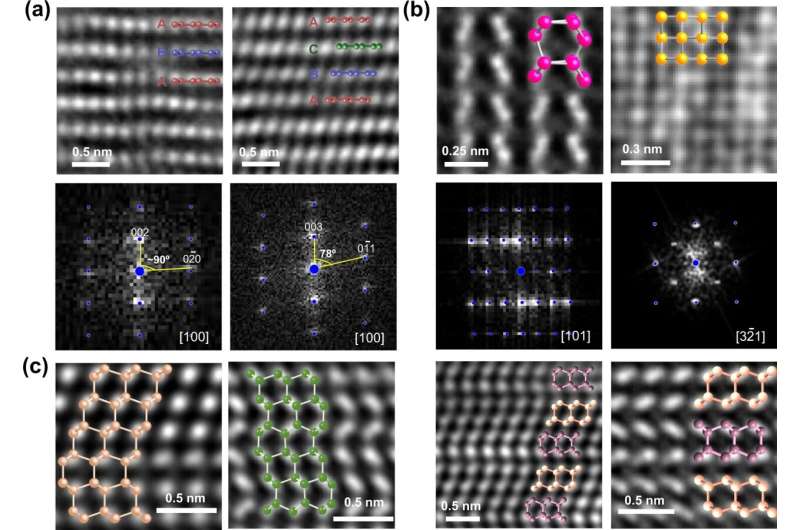
Algorithm review
Scientists trained the ML algorithm using synthetic data generated by simulations that represent approximate results that scientists would obtain through experiments. They generated the dataset using molecular dynamics and density functional theory, both common tools in computational chemistry.
The training data was created using Carbon, a high-performance computing cluster at the Argonne Center for Nanoscale Materials (CNM), a user facility of the DOE Office of Science. Two other DOE user facilities were also used: the Argonne Leadership Computing Facility and the National Energy Research Scientific Computing Center at Lawrence Berkeley National Laboratory.
Using the algorithm’s predictions as a guide, the team verified its effectiveness by synthesizing actual samples and characterizing them with a transmission electron microscope at the CNM.
The algorithm successfully predicted known phase diagrams for carbon, and the computer-generated phase diagrams confirmed and shed light on several unresolved experimental observations.
In particular, the algorithm identified the previously ambiguous structure of n-diamond (standing for “new diamond”), a carbon state that has puzzled scientists since it was theorized over 30 years ago. “The algorithm has made new and surprising predictions about structural features of n-diamonds, which we have verified with experiments, showing that the algorithm holds up even at high-grade phases,” Wen said.
The team also synthesized several algorithm-predicted phases that have not yet been reported in the scientific literature. The structures of the samples matched the predictions, further verifying the algorithm.
“The synthesis of materials, particularly materials with exotic properties, can often require multiple experimental attempts and years of effort,” said Argonne scientist Subramanian Sankaranarayanan, a lead author of the study. “Our machine learning algorithms allow us to identify the synthesis conditions of exotic materials, potentially shortening the time for their experimental implementation.”
In this study, the algorithm was only applied to carbon. In the future, scientists hope to apply the same approach to systems with more than one element. Applying the machine learning algorithm to more complex systems could have far-reaching implications for the discovery and design of useful materials.
An article on the study “Machine learning the metastable phase diagram of covalently bonded carbon” was published in nature communication.
A model trained to predict spectroscopic profiles helps decipher the structure of materials
Srilok Srinivasan et al, Machine learning of the metastable phase diagram of covalently bound carbon, nature communication (2022). DOI: 10.1038/s41467-022-30820-8
Provided by Argonne National Laboratory
Citation: Scientists use Machine Learning to Accelerate Materials Discovery (2022, October 5), retrieved October 5, 2022 from https://phys.org/news/2022-10-scientists-machine-materials-discovery.html
This document is protected by copyright. Except for fair trade for the purpose of private study or research, no part may be reproduced without written permission. The content is for informational purposes only.
#Scientists #machine #learning #accelerate #material #discovery
Leave a Comment