Peek inside the HERA tunnel: Berkeley Lab scientists have developed new machine learning algorithms to speed up the analysis of data collected decades ago by HERA, the world’s most powerful electron-proton collider, which operated from 1992 to 2007 on Aug national research center DESY in Germany. Photo credit: DESY
Protons are tiny but have a lot of weight. They inhabit the center of every atom in the universe and play a crucial role in one of the most powerful forces in nature.
And yet protons also have a down-to-earth side.
Like most particles, protons have a spin that acts like tiny magnets. Flipping a proton’s spin or polarity might sound like science fiction, but it’s the basis for technological breakthroughs that have become essential to our daily lives, such as B. Magnetic Resonance Imaging (MRI), the invaluable medical diagnostic tool.
Despite these advances, the inner workings of the proton remain a mystery.
“Basically everything around us exists because of protons – and yet we still don’t understand everything about them. A big mystery physicists are trying to solve is the spin of the proton,” said Ben Nachman, a physicist who leads the machine learning group in the physics department at the Department of Energy’s Lawrence Berkeley National Laboratory (Berkeley Lab).
Understanding how and why protons spin could lead to technological advances we can’t even imagine today and help us understand the strong force, a fundamental property that gives mass to all protons, and therefore atoms.
But it is not such a simple problem to solve. For one thing, you can’t exactly pick up a proton and place it in a Petri dish: protons are unfathomably small—their radius is a hair’s quadrillionth of a meter, and visible light passes right through them. Also, you can’t see their insides with even the most powerful electron microscopes in the world.
Recent work by Nachman and his team may bring us closer to solving this puzzling proton puzzle.
As a member of the H1 Collaboration – an international group that now includes 150 scientists from 50 institutes and 15 countries and is based at the national research center DESY in Germany – Nachman has developed new algorithms for machine learning to accelerate the analysis of the collected data decades ago by HERA, the world’s most powerful electron-proton accelerator, which ran at DESY from 1992 to 2007.
HERA – a ring four miles in circumference – worked like a giant microscope, accelerating both electrons and protons to nearly the speed of light. The particles collided head-on, allowing a proton to scatter into its components: quarks and gluons.
HERA scientists took measurements of the particle debris cascaded during these electron-proton collisions, what physicists call “deep inelastic scattering,” through sophisticated cameras called particle detectors, one of which was the H1 detector.
Reveal secrets of powerful force
In 2007, the year HERA was decommissioned, the H1 stopped collecting data. The H1 Collaboration still analyzes the data today and publishes the results in scientific journals.
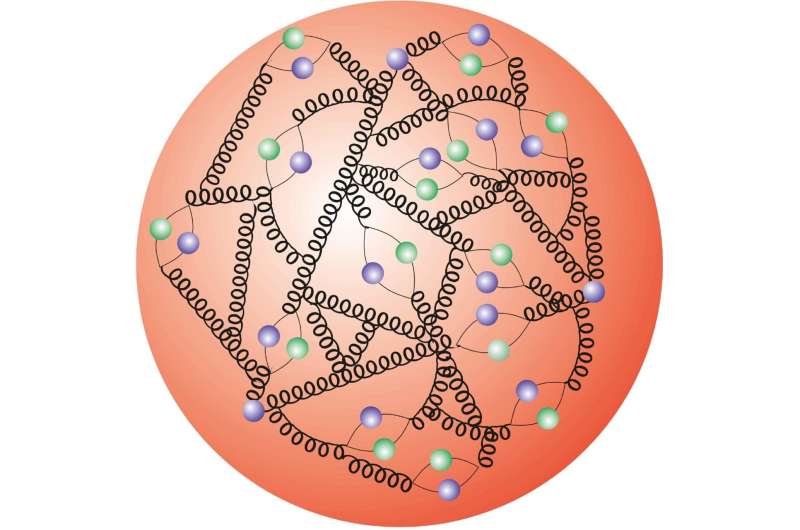
The electron-proton collider HERA accelerated both electrons and protons to almost the speed of light. The particles were collided head-on, allowing a proton to scatter into its constituent parts: quarks (shown as green and purple spheres in the figure above) and gluons (shown as black coils). Photo credit: DESY
It can take a year or more when using conventional computational techniques to measure quantities related to proton structure and strong force, e.g. B. how many particles are produced when a proton collides with an electron.
And if a researcher wanted to study a different quantity, such as how fast particles travel in the wake of a quark-gluon jet stream, they would have to start the long calculation process all over again and wait another year.
A new machine learning tool called OmniFold — co-developed by Nachman — can measure many quantities simultaneously, reducing the time it takes to perform an analysis from years to minutes.
OmniFold does this by instantly using neural networks to combine computer simulations with data. (A neural network is a machine learning tool that processes complex data that scientists cannot do manually.)
Nachman and his team first applied OmniFold to H1 experimental data in a June issue of the journal Physical Verification Letters and more recently at the Deep Inelastic Scattering (DIS) Conference 2022.
To develop OmniFold and test its robustness to H1 data, Nachman and Vinicius Mikuni, a postdoctoral researcher in the Data and Analytics Services (DAS) group at the National Energy Research Scientific Computing Center (NERSC) of Berkeley Lab and a NERSC Exascale Science Applications Program for Learning colleague needed a supercomputer with lots of powerful GPUs (graphics processing units), Nachman said.
Coincidentally, Perlmutter, a new supercomputer designed to support simulations, data analysis, and artificial intelligence experiments that require multiple GPUs simultaneously, had just opened up for an “early science phase” in the summer of 2021, allowing scientists to test the system against real data. (The Perlmutter supercomputer is named after Berkeley Lab cosmologist and Nobel laureate Saul Perlmutter.)
“Because the Perlmutter supercomputer allowed us to use 128 GPUs simultaneously, we were able to complete all steps of the analysis, from data processing to deriving the results, in less than a week instead of months. This improvement allows us to be quick to optimize the neural networks we train and get a more accurate result for the observables we measure,” said Mikuni, who is also a member of the H1 Collaboration.
A central task in these measurements is the consideration of detector distortions. Standing like a sentinel at the entrance to a packed concert hall, the H1 detector monitors particles as they fly through. One source of measurement error occurs, for example, when particles fly around the detector instead of through it – similar to how an unticketed concert-goer jumps over an unguarded fence instead of entering through the ticketed security gate.
A simultaneous correction of all distortions was not possible due to the limited calculation methods available at the time. “Our understanding of subatomic physics and data analysis techniques has evolved significantly since 2007, and scientists can now use new insights to analyze the H1 data,” Nachman said.
Scientists today have a renewed interest in HERA’s particle experiments, as they hope to use the data – and more accurate computer simulations backed by tools like OmniFold – to help analyze the results of future electron-proton experiments, such as on Department of Energy’s next-generation Electron-Ion Collider (EIC).
Planned to be built at Brookhaven National Laboratory in partnership with the Thomas Jefferson National Accelerator Facility, the EIC will be a powerful and versatile new machine capable of accelerating high-energy beams of polarized electrons containing a variety of ions (or charged atoms) to collide many energies, including polarized protons and some polarized ions.
“It’s exciting to think that our method could one day help scientists answer unanswered questions about the strong force,” Nachman said.
“While this work may not lead to practical applications in the near future, we are here to understand the building blocks of nature – to seek ultimate truth.” These are steps to understand, at the most basic level, what everything is made of.” . That’s what drives me. If we don’t do the research now, we’ll never know what exciting new technological advances we’ll make to benefit future societies.”
Signs of saturation appear in particle collisions at RHIC
V. Andreev et al, Measuring Lepton Jet Correlation in Deep Inelastic Scattering with the H1 Detector Using Machine Learning for Deconvolution, Physical Verification Letters (2022). DOI: 10.1103/PhysRevLett.128.132002
OmniFold: arxiv.org/abs/1911.09107
Conference presentation: www-h1.desy.de/psfiles/confpap … /H1prelim-22-034.pdf
Provided by Lawrence Berkeley National Laboratory
Citation: How to solve a problem like a proton? Smash it, then build it back with machine learning (2022, October 25), retrieved October 25, 2022 from https://phys.org/news/2022-10-problem-proton-machine.html
This document is protected by copyright. Except for fair trade for the purpose of private study or research, no part may be reproduced without written permission. The content is for informational purposes only.
#solve #problem #proton #Smash #rebuild #machine #learning
Leave a Comment