In a new approach to replicating quantum entanglement, extra “ghost” electrons are controlled by an artificial intelligence technique called a neural network. The network tweaks until it finds an accurate solution that can be projected back into the real world, recreating the effects of entanglement without the attendant computational hurdles. Credit: Lucy Reading-Ikkanda/Simons Foundation
Physicists (temporarily) extend reality to crack the code of quantum systems.
Predicting the properties of a molecule or material requires calculating the collective behavior of its electrons. Such predictions could one day help researchers to develop new medicines or design materials with desirable properties such as superconductivity. The problem is that electrons can “quantum mechanically” become entangled with one another, i.e. they can no longer be treated individually. The tangled web of connections becomes absurdly difficult for even the most powerful computers to untangle directly for any system with more than a handful of particles.
Now, quantum physicists at the Flatiron Institute’s Center for Computational Quantum Physics (CCQ) in New York City and the École Polytechnique Fédérale de Lausanne (EPFL) in Switzerland have circumvented the problem. They created a way to simulate entanglement by adding additional “ghost” electrons to their calculations, which interact with the actual electrons of the system.
In the new approach, the behavior of the added electrons is controlled by an artificial intelligence technique called a neural network. The network tweaks until it finds an accurate solution that can be projected back into the real world, replicating the effects of entanglement without the attendant computational hurdles.
The physicists will present their method on August 3rd Proceedings of the National Academy of Sciences.
“You can treat the electrons as if they are not talking to each other, as if they are not interacting,” says the study’s lead author Javier Robledo Moreno, a graduate student at CCQ and New York University. “The additional particles we add mediate the interactions between the actual particles living in the actual physical system we are trying to describe.”
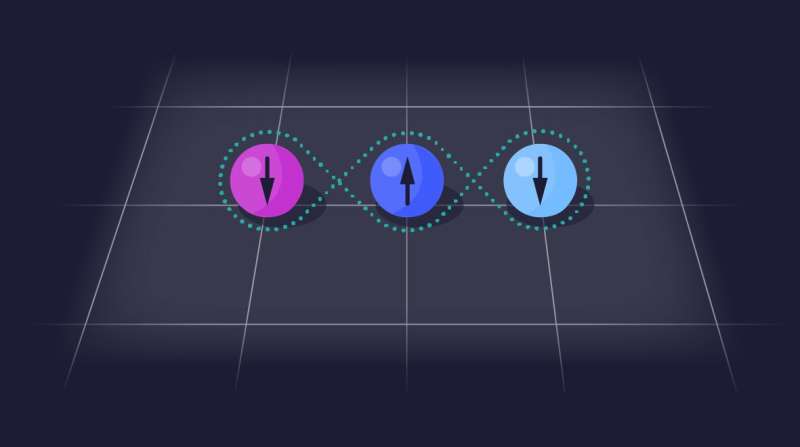
An illustration of quantum entanglement. Credit: Lucy Reading-Ikkanda/Simons Foundation
In the new work, the physicists show that their approach matches or outperforms competing methods in simple quantum systems.
“We applied this to simple things as a test bed, but now we’re going to take it to the next step and try it on molecules and other, more realistic problems,” says Antoine Georges, co-author of the study and CCQ director. “It’s a big deal, because if you get the wavefunctions of complex molecules well, you can do all sorts of things, like design drugs and materials with certain properties.”
The long-term goal, Georges says, is to enable researchers to computationally predict the properties of a material or molecule without having to synthesize it and test it in a lab. For example, they could test a large number of different molecules for a desired pharmaceutical property with just a few mouse clicks. “Simulating large molecules is a big thing,” says Georges.
Robledo Moreno and Georges co-authored the paper with EPFL Assistant Professor of Physics Giuseppe Carleo and CCQ Research Fellow James Stokes.
The new work is an evolution of a 2017 publication Science by Carleo and Matthias Troyer, currently Technical Fellows at Microsoft. This paper also combined neural networks with fictitious particles, but the particles added were not full-blown electrons. Instead, they only had one property known as spin.
“As I was [at the CCQ] in New York, I became obsessed with the idea of finding a version of a neural network that describes how electrons behave, and I was keen to find a generalization of the approach we introduced in 2017,” says Carleo. “With this new one, we finally found an elegant way to have hidden particles that aren’t spins, they’re electrons.”
Team Script’s breakthrough quantum algorithm
Javier Robledo Moreno et al., Fermionic wave functions from hidden states constrained by neural networks, Proceedings of the National Academy of Sciences (2022). DOI: 10.1073/pnas.2122059119
Provided by the Simons Foundation
Citation: Neural Networks and “Ghost” Electrons Accurately Reconstruct the Behavior of Quantum Systems (2022 August 3) retrieved August 3, 2022 from https://phys.org/news/2022-08-neural-networks-ghost- electrons-accurately.html
This document is protected by copyright. Except for fair trade for the purpose of private study or research, no part may be reproduced without written permission. The content is for informational purposes only.
#Neural #networks #ghost #electrons #accurately #reconstruct #behavior #quantum #systems
Leave a Comment